A New Leap in Multimodal Language Models
Welcome to the exciting world of AI research! Today, we’re delving into the groundbreaking study of “Ferret,” a Multimodal Large Language Model (MLLM) that’s redefining how AI understands and interacts with images and text.
Ferret is a game-changer in spatial understanding and localization in images. This model’s unique hybrid region representation, which combines discrete coordinates and continuous visual features, allows for precise handling of various region shapes. The model’s performance, particularly in accurately describing image details, their relationships to otherobjects and reducing object hallucinations, is a significant leap forward.
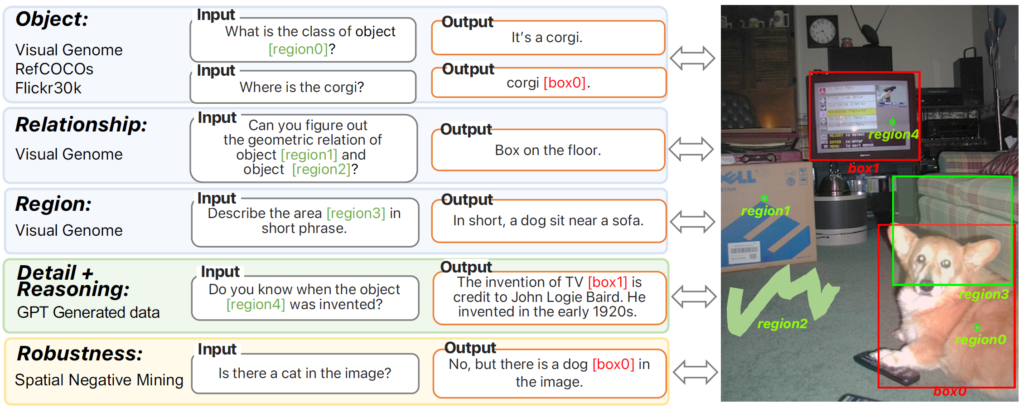
Released by researchers at Apple, the model was trained on a novel GRIT Dataset (~1.1M), A Large-scale, Hierarchical, Robust ground-and-refer instruction tuning dataset. In addition to creating this training set, the authors also invented a benchmarking system called Ferret-Bench. It is a multimodal evaluation benchmark that jointly requires Referring/Grounding, Semantics, Knowledge, and Reasoning
Areas for Future Research
The journey doesn’t end here. Future research aims to enhance Ferret’s spatial understanding, tackle the ambiguity in referring expressions, broaden dataset diversity, integrate with other modalities, and explore real-world applications.
Acknowledged Limitations
However, every innovation comes with its set of challenges. Ferret’s effectiveness depends heavily on the dataset quality. Generalizing to new types of images or spatial relationships poses a challenge. Also, handling ambiguous expressions and the need for substantial computational resources are areas that need attention.
This study presents a fascinating glimpse into the future of AI and multimodal interactions. Stay tuned for more insights as we continue to explore the limitless possibilities of artificial intelligence!
The paper is available here and the code available on Github